How Human Feedback Improves AI Chatbots
Learn how human feedback enhances AI chatbots, improving accuracy, understanding, and customer support efficiency.
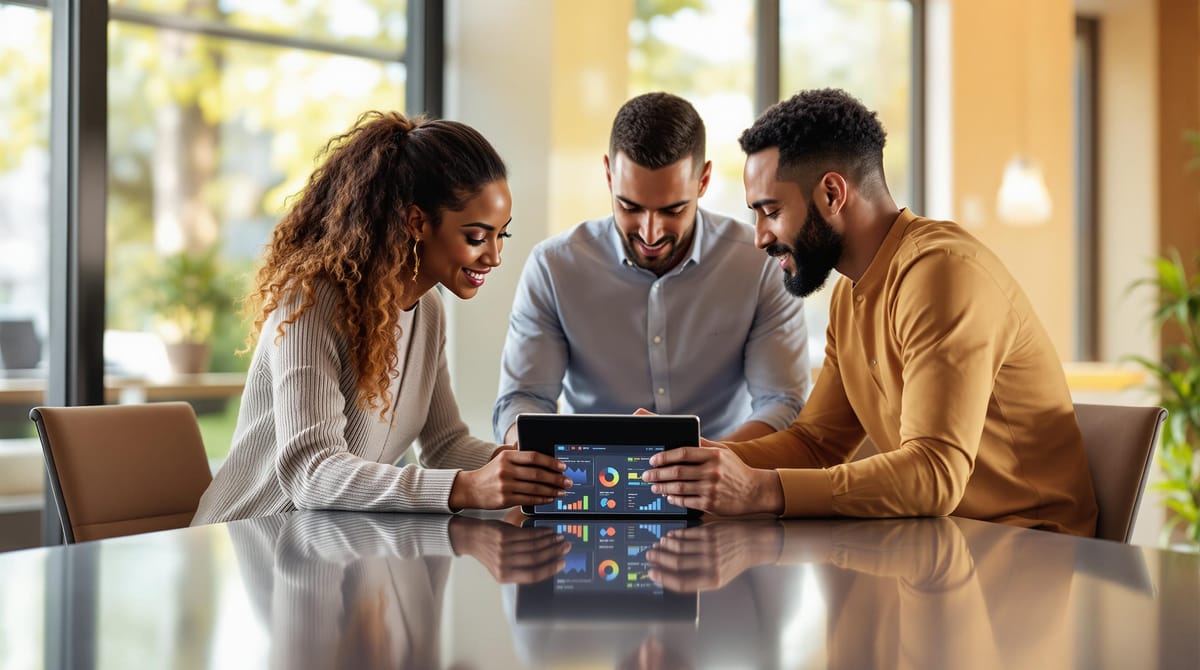
AI chatbots are useful for customer support, but they often fail without human input. Here's how human feedback makes chatbots better:
- Fixing Errors: Agents correct chatbot mistakes, helping them learn and improve.
- Better Understanding: Feedback ensures bots grasp complex questions and emotional cues.
- Updating Knowledge: Teams fill gaps in the chatbot's database to keep responses accurate.
- Consistency: Human input aligns chatbot replies with the brand's tone and style.
Key Feedback Types:
- User Ratings: Thumbs up/down, surveys, and reviews highlight chatbot performance.
- Agent Corrections: Live fixes and training insights refine responses.
- Error Analysis: Identifying frequent escalations and unanswered questions improves accuracy.
By combining automation with continuous feedback, chatbots become smarter, more reliable, and capable of handling real-world interactions effectively.
Main Types of Human Feedback
AI chatbots improve by gathering and learning from three main types of feedback.
User Ratings and Reviews
Ratings and reviews from users provide insights into what’s working and what isn’t. This feedback often comes in forms like:
- Satisfaction ratings: Options like thumbs up/down or star ratings after interactions
- Reaction buttons: Prompts such as "Was this helpful?" or "Did this solve your problem?"
- Follow-up surveys: Short questionnaires asking about the quality of the interaction
For example, if users consistently rate information on shipping policies poorly, it’s a sign that this area needs updating to be clearer and more useful.
Agent Response Corrections
Support agents play a crucial role in refining chatbot responses. They address inaccuracies by:
- Making immediate corrections: Flagging incorrect answers during live conversation takeovers
- Improving phrasing: Suggesting better ways to word responses or handle specific queries
- Providing training insights: Documenting why certain responses didn’t meet user needs
These corrections are used as training data to help the chatbot respond more effectively in the future. By studying why interactions fail, teams can identify gaps in the bot's knowledge.
Identifying Knowledge Gaps
Systematic error analysis is another essential feedback method. It focuses on patterns that reveal where the chatbot struggles, such as:
- Escalation patterns: Topics frequently handed off to human agents
- Unanswered questions: Instances where the bot responds with "I don't understand"
- Abandoned chats: Conversations that end without resolution
By analyzing these issues, support teams can:
- Expand the bot’s knowledge base
- Create new training scenarios
- Fine-tune responses for better accuracy
This approach not only improves the chatbot's performance but also minimizes future misunderstandings, ensuring consistent and reliable support.
Making Chatbots Better Through Feedback Cycles
Using Chat Data to Improve AI
Reviewing chat transcripts can sharpen how AI understands and responds. The main areas to analyze include:
- Intent accuracy: Is the bot correctly interpreting user goals?
- Relevance of responses: Are the answers helpful and on point?
- Conversation flow: Does the interaction feel natural and efficient?
- Language patterns: What common phrases or terms do users rely on?
This analysis helps fine-tune the chatbot to better align with your business needs.
Tailoring Chatbots to Fit Your Business
To make a chatbot truly useful, it needs to reflect your business's unique characteristics. This can be achieved by focusing on:
- Industry terminology: Teach the bot to handle specialized language.
- Brand voice: Make sure the bot communicates in a way that matches your company’s tone.
- Product knowledge: Equip the bot with accurate details about your products or services.
- Support protocols: Ensure it follows your customer service standards.
The goal is for the chatbot to feel like an extension of your support team, delivering responses that are as reliable as those from a human agent.
Real-World Feedback Improvements
Practical feedback loops are key to improving chatbot performance. Here’s how they work:
-
Improving Response Accuracy
Responses flagged as unhelpful are identified and reviewed. This allows you to update the bot’s knowledge base with better information. -
Streamlining Conversation Flow
By studying successful interactions, you can pinpoint dialogue patterns that guide users to solutions more effectively. -
Boosting Context Awareness
Over time, the bot learns to distinguish between different types of queries, like order tracking versus general policy questions.
Every interaction offers insights that can make the chatbot smarter and more efficient. Regularly reviewing feedback ensures the bot keeps meeting user expectations while lightening the load on your support team.
HelpJam's Human Feedback Features
HelpJam uses built-in tools to fine-tune chatbot performance by blending automated responses with ongoing learning. This approach ensures more accurate support over time.
Agent Takeover Learning
When agents step in to correct a chatbot's response, HelpJam treats these moments as learning opportunities. The AI engine reviews:
- Response accuracy: How closely the bot's answer aligned with the correct solution.
- Context recognition: Whether the bot understood the customer's intent correctly.
- Solution effectiveness: If the response fully addressed the issue.
Every correction becomes part of the bot's training data, reducing the chance of repeating similar errors. This process also highlights areas where the bot needs more training.
Customer Feedback Tools
HelpJam collects and analyzes customer feedback using various tools:
- Instant reaction buttons for quick ratings.
- Post-conversation surveys to gauge interaction quality.
- An analytics dashboard to monitor satisfaction trends.
- Sentiment analysis to pinpoint areas for improvement.
By consolidating this feedback, HelpJam provides actionable insights. Support teams can easily identify which queries perform well and which need attention, enabling targeted updates.
Simple Training Updates
Enhancing your chatbot’s knowledge is straightforward with HelpJam, no technical skills required:
- One-click updates to add new information seamlessly.
- A visual interface for organizing training content.
- Bulk editing to update multiple responses at once.
This no-code setup allows support teams to refine chatbot responses based on real-world interactions, ensuring accuracy while minimizing the need for agent involvement.
Key performance metrics are accessible through the analytics dashboard:
Metric | Description |
---|---|
Resolution Rate | Percentage of queries handled by the bot. |
Learning Efficiency | Speed at which training data is applied. |
Customer Satisfaction | Average rating of bot responses. |
Escalation Reduction | Fewer cases requiring human intervention. |
These tools and features create a dynamic support system that learns and improves with every interaction, all while being simple for teams to manage.
Conclusion: Better Chatbots Through Human Input
Main Points
Human feedback turns AI chatbots into smarter, more reliable tools for customer support. Here's how this feedback loop helps:
- Improved Accuracy: Chatbots learn from actual customer interactions and agent corrections, cutting down on future mistakes.
- Stronger Context Understanding: Bots get better at grasping what customers mean, even with complex requests.
- Personalized Support: Human input ensures responses stay aligned with the brand's tone and meet customer expectations.
These advancements highlight the foundation of HelpJam's approach.
HelpJam's Advantages
HelpJam takes these benefits further, offering tools and systems to refine chatbots effectively:
- Smart Learning System: Uses feedback like corrections and ratings to fine-tune responses.
- Real-Time Analytics: Monitors performance with metrics such as resolution rates and customer satisfaction.
- No-Code Updates: Allows teams to update chatbot knowledge easily, no technical skills needed.
By blending automation with human insights, businesses can deliver stronger customer support while easing the burden on their teams.
Performance Area | Impact |
---|---|
Response Quality | More accurate and relevant answers |
Customer Experience | Conversations feel more natural |
Support Efficiency | Less need for agent involvement |
Knowledge Base | Content stays updated and relevant |